Limited Memory AI: How Machines Learn from the Past
Limited Memory AI: How Machines Learn from the Past
When we think of artificial intelligence (AI), the idea of machines learning from past experiences to improve their future actions comes to mind. This capability is known as Limited Memory AI, a form of AI that uses historical data and previous experiences to make more informed decisions. Unlike reactive machines, which only respond to immediate inputs, limited memory AI systems are able to “remember” and adapt, giving them a much higher level of functionality.
In this article, we’ll explore how Limited Memory AI works, how it differs from reactive AI, and where it’s applied in the real world. From self-driving cars to fraud detection, we’ll examine the benefits and challenges of this technology.
What is Limited Memory AI?
At its core, Limited Memory AI refers to systems that store and learn from historical data to enhance future decision-making. These systems are built to retain information from past interactions and experiences, allowing them to improve their accuracy and functionality over time.
How It Works
Limited Memory AI systems rely on datasets collected over time to build a model of the world. They don’t simply react to real-time inputs like reactive machines; instead, they use a combination of current data and past experiences to make decisions. For example, an autonomous vehicle may use data from previous trips, combined with real-time sensor information, to navigate a road more efficiently.
Key Features:
- Memory-based decision-making: Stores past data to improve performance in future tasks.
- Learning from experience: Continually adapts based on new data.
- Improved accuracy: Able to refine decisions based on historical context.
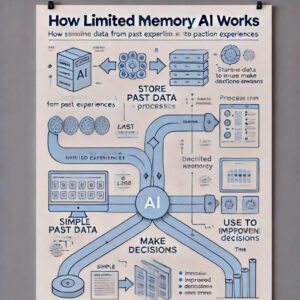
How It Differs from Reactive AI
While both reactive AI and limited memory AI serve important roles, their fundamental difference lies in how they process information. Reactive AI makes decisions solely based on the current situation. It doesn’t learn or store data, meaning each decision is made in isolation, as if it’s encountering the task for the first time every time.
Comparison of Reactive AI vs. Limited Memory AI
Feature | Reactive AI | Limited Memory AI |
---|---|---|
Memory | No memory, no learning from past data | Stores past experiences to improve over time |
Decision-making process | Real-time reactions based on current input | Uses both current and historical data |
Adaptability | Cannot adapt to new situations | Adapts and learns from past data |
Typical applications | Chess-playing AI, factory robots | Self-driving cars, fraud detection, NLP models |
Applications of Limited Memory AI
Limited memory AI is used in a wide range of industries, especially where learning from data is essential for improving future outcomes. Here are some key applications:
Autonomous Vehicles
Self-driving cars rely heavily on limited memory AI to navigate roads. These systems use data from previous trips to recognize traffic patterns, road conditions, and potential hazards. Tesla’s self-driving cars are a great example of this, using cameras and sensors to continuously learn and improve their driving algorithms.
Example: Tesla’s Self-Driving AI Tesla vehicles use AI that combines real-time data with historical information about roads, traffic, and driving conditions. By analyzing past experiences, these vehicles can better anticipate and avoid obstacles, making the driving experience safer and more efficient.
Fraud Detection Systems
Banks and financial institutions use limited memory AI to detect fraudulent activities. These systems analyze past transactions to identify patterns of fraud, then use this historical data to flag suspicious behavior in real-time. This helps prevent fraud by learning from past mistakes and continuously improving the detection process.
Stock Market Prediction Models
Stock market prediction models rely on massive amounts of historical data to predict future trends. These models use limited memory AI to analyze patterns in past stock prices, trading volumes, and economic data. Based on these patterns, the AI can make informed predictions about future market movements.
Challenges and Limitations of Limited Memory AI
Despite its ability to learn from past data, Limited Memory AI has its own set of challenges and limitations. While it provides a higher level of functionality than reactive AI, it’s not without flaws.
Limited Ability to Generalize Data
One of the key limitations of limited memory AI is its struggle to generalize data across different scenarios. The system learns from specific datasets, which means its predictions are only as good as the data it has been trained on. If the AI encounters a new situation that doesn’t match its training data, it may struggle to make accurate decisions.
Potential for Bias
Since limited memory AI relies on historical data, it can sometimes reinforce biases present in that data. For example, if a fraud detection system is trained on data that contains biased patterns, it may continue to make biased decisions in the future.
Computational Requirements
Limited memory AI systems require substantial computational power to process and store vast amounts of historical data. This can be a barrier for some organizations, particularly when dealing with large datasets or complex models.
Examples of Limited Memory AI
Limited memory AI systems are becoming increasingly common, particularly in areas where learning from the past is critical to success. Here are some examples of how this AI is used in the real world.
Tesla’s Self-Driving AI
Tesla’s autonomous vehicles are perhaps the most well-known example of limited memory AI. These cars use a combination of real-time data and stored experiences to navigate roads, making adjustments based on both current traffic conditions and past driving experiences.
Fraud Detection Algorithms
Banks and credit card companies use AI-powered fraud detection systems that analyze past transactions to identify patterns of fraud. By learning from historical data, these systems can detect suspicious activity and prevent fraud more effectively.
Natural Language Processing (NLP) Models
Some NLP models, such as those used in virtual assistants like Siri or Alexa, rely on limited memory AI to improve their language understanding over time. By analyzing previous interactions, these systems can improve their ability to understand and respond to user queries.
Conclusion
Limited memory AI represents a significant step forward from reactive AI, allowing machines to learn from the past and improve their decision-making abilities over time. While these systems come with their own set of challenges—such as computational requirements and potential biases—their applications in industries like transportation, finance, and language processing are undeniable.
As AI continues to evolve, limited memory systems will likely play an even greater role in shaping the future of autonomous vehicles, fraud prevention, and data-driven decision-making.
Post Comment